Why image using volume electron microscopy?
Life occurs in 3 dimensions, therefore it is of utmost importance to also investigate life in 3D. Only in 3D, we can study the varied shapes of organelles in different cell types and states of cellular health. With the development of volume electron microscopy (volume-EM), researchers can image biological tissue and obtain 3D images at a much higher resolution than in light microscopy. In these images, the ultrastructure of the tissue can be viewed, which is especially important for structural cell biology, and experimental and diagnostic pathology [1][2].
The general workflow of volume-EM is as follows: a sample is (i) embedded in resin, (ii) sectioned into small slices (Figure 1), (iii) imaged using a transmission or scanning electron microscope, and then (iv) the images are stacked into a 3D dataset and analyzed. When analyzing the volume-EM images, one of the most crucial first steps is to perform segmentation of an object of interest, such as a cell organelle. Executing this manually is time-consuming, especially for imaging large volumes, so automation is needed.
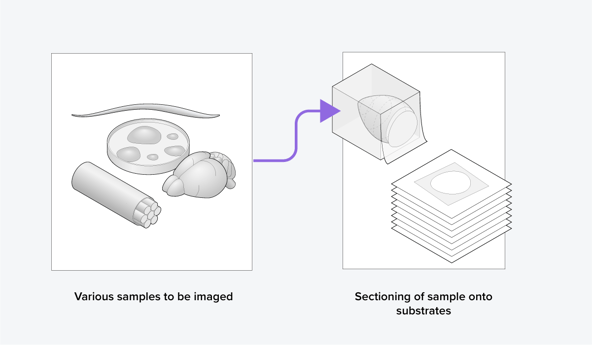
Figure 1: Samples are sectioned as part of the volume electron microscopy workflow.
How can image segmentation of organelles be automated?
Automation can be done using artificial intelligence (AI). For the segmentation of organelles in volume-EM images, a subfield of AI called deep learning (DL) has been adopted recently [3]. To create a volume-EM segmentation algorithm, a large dataset is always needed for training the model. The DL model is then usually trained on 3D information about the features of the organelle. It can in this way learn to recognize the organelle that has to be segmented from the examples in the training dataset. Then, it is evaluated using the test dataset, in which it has to segment the organelle. The model is tuned and subjected to iterations of training-testing-tuning so that the model can automatically segment the organelle in the subsequently given images successfully.
A previous bottleneck was that it used to be quite hard to find AI models in general that performed well at segmentation tasks. Fortunately, a repository called BioImage.io facilitates this by providing an overview of the available AI image analysis models and training data, for researchers in the field of biology. The repository also includes segmentation algorithms and training data sets for volume-EM.
Another downside of these deep learning algorithms was that they typically could only perform segmentation on images of one cell type and one imaging mode. There were no general algorithms to segment organelles such as mitochondria from different cell types. Mitochondria vastly differ per cell type and an altered morphology is often associated with mitochondrial dysfunctions, which are implicated in many diseases [4][5]. To better characterize these mitochondrial dysfunctions, Volume-EM can be a great tool for imaging them in tissue with a resolution high enough to analyze their morphological changes.
How have recent efforts facilitated organelle segmentation in multiple cell types?
Recently, a model was developed that can segment mitochondria located in cells that it was not trained on. This model by Conrad et al. [5] was trained on 2D images obtained from 3D volume-EM data. The researchers formed a large dataset of 2D mitochondrial images with various cellular contexts called CEM-MitoLab. They used part of this as a training dataset to train Panoptic-DeepLab (PDL) models for segmentation.
The best-performing model, named MitoNet, could accurately segment mitochondria in 2D and 3D in various cellular contexts. After training MitoNet specifically on the salivary gland images, which are often hard to segment, it also performed well. Therefore, the model can be trained on a specific cellular context to improve the segmentation accuracy even more, a process called transfer learning. They found that training the model on heterogeneous datasets was more successful than training on large(r) homogeneous datasets.
This generalized segmentation tool can make automated image analysis more accessible to biologists. When more of these generalized methods are developed, this can enable biologists to perform quantitative analysis on volume-EM images of organelles in various cellular contexts. The imaging throughput of conventional electron microscopes is however quite low, therefore it can take years to obtain these EM images of organelles in different cellular contexts [6].
How can the throughput of volume-EM be further increased?
Recently, Delmic developed the FAST-EM, an ultrafast scanning transmission electron microscope (STEM) that images using 64 electron beams in parallel. Together with its optimized data management method, up to 100 times faster imaging speed can be achieved over conventional scanning electron microscopes (SEM) using only one beam for imaging. With FAST-EM, a large volume-EM dataset can thus be obtained in a short time span by imaging consecutive images, making it an ideal volume-EM imaging tool (Figure 2). This enables the collection of a heterogeneous organelle dataset for training novel generalized segmentation models, leading to faster and more streamlined data analysis for the biosciences community.
Figure 2: Using the FAST-EM, training datasets can be easily made to train a deep learning model to segment organelles automatically in various cellular contexts.
FAST-EM furthermore gives the opportunity to image larger areas, such as a whole brain or a large tissue sample, and perform comparative studies at a high resolution. A large dataset of volume-EM images can subsequently be analyzed using (1) automated segmentation algorithms and (2) image analysis platforms. From this, a large set of quantitative and qualitative biological data can be obtained, leading to more statistically sound data (Figure 3). This could make results more publishable and overall strengthen biological research.
Figure 3: After automated segmentation, further data analysis can reveal quantitative information on the organelles of interest.
Conclusion
Generalized segmentation models such as the MitoNet thus open possibilities for the development of generalized segmentation models for other organelles in volume-EM data. The availability of organelle segmentation models that can be applied to different cell types lowers the barrier of volume-EM data analysis in biological research. In combination with our FAST-EM, large volumes of tissue could be imaged and quantitatively analyzed in a short time span. We think this could revolutionize the way biological research is done.
References
[1] B. Titze and C. Genoud, Biology of the Cell 108, 11, (2016)
[2] Kuwajima et al., Neuroscience 251,75–89, (2013)
[3] Kievits et al., J Microsc 287,3, 114–137, (2022)
[4] T. Doke and K. Susztak, Trends Cell Biol 32, 10, 841–853, (2022)
[5] Siegmund et al., iScience 6, 83-91, (2018)
[6] R. Conrad and K. Narayan, bioRxiv preprint, (2022)
[7] Radulović et al., Histochem Cell Biol 158, 3, 203–211, (2022)